课程名称 |
讲授教师 |
课程简介 |
视频地址 (请使用超星客户端扫描课程二维码) |
Introduction to Latent Variable Modeling (潜变量模型) |
Fan Y. Wallentin Professor of Statistics at Uppsala University, Sweden |
Thecourse introduces the core methods of latent variable modeling (LVM), including structural equation models (SEM) and Latent growth models (LGM), which uses in the structural equation modeling framework to estimate growth trajectories. These are a family of statistical approaches that explore complex relationships between and among latent (unobserved) and observed variables, as well as the longitudinal analysis technique to estimate growth over a period of time. The course will cover LVM with cross-sectional data in single and multiple-group settings and the methodology of LGM to investigate systematic change and growth and inter-individual variability in the change. The course emphasizes the empirical applications of LVM and LGM techniques to broadly address relevant questions in the different disciplines. Course lectures, readings, and assignments will reflect this applied focus. At the end of the course, the student is expected to understand the essential issues within SEM and LGM. The students should be able to carry out analysis independently. /info/1037/4091.htm |
邀请码: 67758900 手机APP首页右上角输入 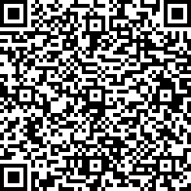
|
Time series forecasting (时间序列预测) |
Fotios Petropoulos Professor of Management Science at the School of Management of the University of Bath |
This unit will introduce you to concepts of data analysis using time series forecasting techniques. You will analyse and interpret the patterns in a set of given data, estimate the future observations of data using the trends identified, and use software for statistical analysis and forecasting. This course is suitable for students who are interesting in predictive analytics and have a strong background in statistics and economics. Also, basic knowledge of programming is necessary, as the practical part of the course will be delivered using the R statistical software. /info/1037/3840.htm |
邀请码: 43592772 手机APP首页右上角输入 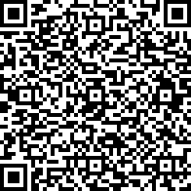
|
Bayesian Statistical Learning (贝叶斯统计学习) |
Matias Quirozis School of Mathematical and Physical Sciences at the University of Technology Sydney. |
The Bayesian approach to statistical inference has gained widespread use in Statistics and Machine Learning. This course aims to provide a solid introduction to Bayesian data analysis via a mixture of theoretical and methodological concepts, with an emphasis in computer implementation of modern simulation algorithms. The course will be presented through four modules (see details below). The first module introduces the Bayesian paradigm and develops inferential tools for some simple models. The second module considers more advanced models, such as linear regression, spline regression and classification models. The third module focuses on computation and presents state-of-the-art algorithms to carry out Bayesian inference. Finally, the fourth module presents model comparison techniques and advanced topics such as Bayesian variable selection and hierarchical models. In each module, the students will be presented the underlying theory and methodology, which will then be demonstrated through an assignment the students will carry on individually. The computer lab session will then discuss possible solutions. Students will gain knowledge in modern state-of-the-art Bayesian methods that can be applied to solve complex problems in Statistics and Machine Learning. The course puts a lot of emphasis on computer implementation of the presented material. The recommended programming language to solve the computer labs is R because the material presented will use it. However, students may use any software they want (e.g. Python or Julia). /info/1037/3807.htm |
邀请码: 24992841 手机APP首页右上角输入 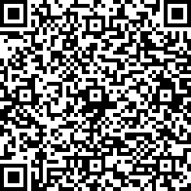
|
Statistical Machine Learning (统计机器学习) |
Minh-Ngoc Tran Associate Professor at University of Sydney Business School |
Data Science is an emerging and inherently interdisciplinary field, with Statistical Machine Learning techniques forming a key set of skills. This course will cover a wide range of popular supervised and unsupervised learning methods including regression, classification, deep learning, model selection and Bayesian computation. The course will also offer an insight into how these statistical methodologies are applied into business applications such as credit scoring and customer behavior analysis. /info/1037/4003.htm |
邀请码: 60808739 手机APP首页右上角输入 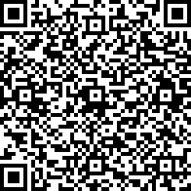
|
Dimension Reductionand Manifold Learning (降维与流形学习) |
Anastasios Panagiotelis Associate Professor of Business Analytics at the University of Sydney Business School |
Many modern datasets contain a large number of variables, that is they are high dimensional. Such high dimensional datasets are often however characterised by an underlying low dimensional structure. Recovering this low dimensional structure can enable exploratory analysis, visualisation and feature construction. This course takes a journey through the development of dimensionality reduction techniques. They range from linear methods such as Principal Components Analysis and Classical Multidimensional Scaling through to modern Manifold Learning techniques includingIsomap,Laplacian Eigenmaps and Local Linear Embedding. Also covered will be the evaluation of different dimension reduction techniques compared to one another, both with respect to computational considerations and with respect to the fidelity with which they capture the structure of high dimensional data. Applications of dimensionality data to real data problems are also a integral part of this course. /info/1037/4092.htm |
邀请码: 82167802 手机APP首页右上角输入 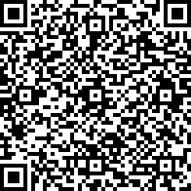
|